About Me
Hi, I am Peng Jiang, a PhD student in Tsinghua University, Beijing, China.
My research interests include multitask learning & lifelong learning, task compositionality & generalization, neural representation & representation alignment, brain & LLM, and mechanistic interpretability.
I am curious about how can the intelligent systems (brain & LLM) can retain learned knowledge from previous tasks and use it to facilitate future learning. To further understand this, I am currently working on studying task representation from the perspective of task compositionality, which I believe is a promising approach to explain generalization. Additionally, I hope to discover the similar underlying mechanisms of multitask representation in brain & LLM.
Education
Tsinghua University
PhD candicate, Computational Neuroscience
2021 - now
At the neural coding lab, we seek to understand how information is represented and processed in the brain to support flexible perception and behavior. Our research lies at the intersection of neuroscience, machine learning and psychology.
I am supervised by Prof. Xiaoxuan Jia in Neural coding lab. In Jiaxx lab, I have explored different topics: visual coding, dimensionality reduction, neural dynamics, communication subspace across brain areas, RNN etc. Now I’m focusing on understanding multitask representation in the view of task compositionality in different learning systems (brain & LLM).
Tsinghua University
BSc, Major in Life Sciences, Minor in Computer Science
2017 - 2021
In Wei Zhang lab, I studied neural mechanism of social space behaviour of drosophila and tried to develop a new tools for inhibiting function of single synapse.
In Bo Hong lab, I completed my undergraduate thesis project, “Construct spiking neural network model for information communication between the hippocampus and the temporal lobe”.
Projects
Task Representation Project
Discover the essence of intelligence, abstraction and generalization.
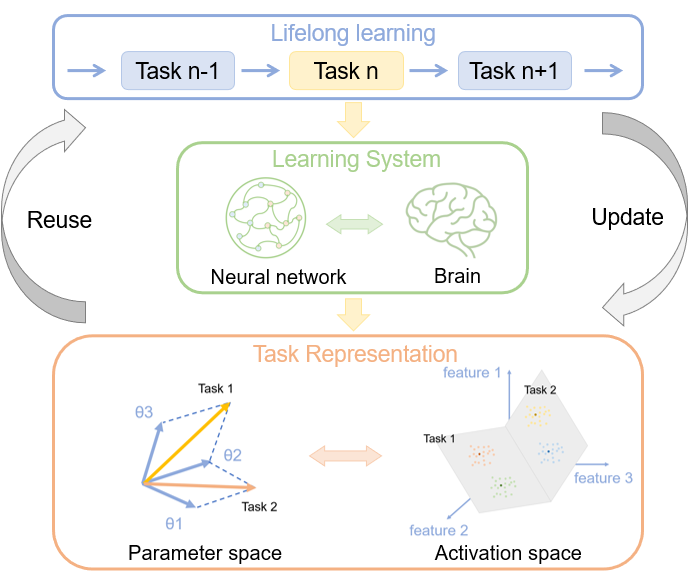
To understand the updating and reuse of knowledge in lifelong learning, it is essential to grasp how learning systems represent the relatedness of tasks. The foundational ideas include:
- Multitask representation can be decomposed into sparse, independent features, with each task represented by a combination of different features.
- Upon learning a new task, the system may utilize existing sparse features or introduce new ones.
Further topics include:
- In the brain
- Does the brain utilize a similar mechanism to represent task relatedness?
- Is there a communication subspace spanned by shared task features across brain areas for information transfer?
- In LLM:
- Do LLMs utilize a similar mechanism to represent task relatedness?
- How can we bridge multitask representation between the parameter space and activation space?
Experience
Neural Match Academy
Summer School
-
Computational Neuroscience July 2022
- I have learned the basic knowledge of computational neuroscience and how to use it to understand the brain. Besides, we have a group project to study “Hidden state dynamics in visual cortex and motor cortex using steinmetz dataset”.
-
Deep learning July 2023
- I have learned the basic knowledge of deep learning and how to use it to solve real world problems. Besides, we have a group project to study “Exploring representational similarity between brain and LLM”.
Neural Coding Lab
Computational Neuroscience
- Explore Visual Coding - Neuropixels dataset from Allen Institute to study neural dynamics and information flow across brain areas.
- Construct multi-areas RNN model for multiple tasks learning to study hierarchy processing of task representation for flexible computation.
Some Other Intersts
![]() |
|